A prediction method of karst cave scale based on the binary classification model of the Gaussian process
-
摘要: 溶洞规模与其影响因素之间存在着复杂的非线性关系,如何根据影响因素有效预测溶洞规模是一类复杂的模式识别问题。基于高斯过程二元分类模型,提出一种溶洞规模的预测方法,该方法通过对样本的学习,建立溶洞规模与其影响因素之间复杂的非线性映射关系,对仅提供影响因素的预测样本进行具有概率意义的识别输出。研究结果表明,该方法除具有小样本、模型参数自适应确定、识别精度高等优点之外,还能够对预测结果给予概率意义的可信度,为实际工程有效预测溶洞规模提供了定量的依据,具有良好的应用前景。Abstract: A complex non-linear relationship exists between the scale of karst caves and its influencing factors. While the scale of karst caves can be predicted by pattern recognition based on influencing factors. A method based on the Gaussian process for the binary classification model (GPC) is proposed to predict the scale of karst caves. In this method, the complex nonlinear relationship between the scale of karst caves and influencing factors is established by learning a few samples. It gives probabilistic output identification for forecasting samples that only provide influencing factors. Research suggests that the proposed method not only has merits of small training samples, self-adaptive parameters determination and high recognition accuracy, but also can give the probabilistic credibility for prediction results. This method can provide a quantitative basis for effective prediction of the scale of karst caves in engineering practice, and has a good application prospect.
-
Key words:
- scale of karst cave /
- prediction /
- Gaussian process /
- binary classification model /
-
[1] 王增银,万军伟,姚长宏.清江流域溶洞发育特征[J].中国岩溶,1999(2):51-58. [2] 何翊武,傅鹤林,罗立峰,等.隧底岩溶洞对结构稳定性的理论解 [J].土木工程学报,2014,47(10):128-135. [3] 徐善初,陈建平,左昌群,等.模糊综合评判法在隧道施工岩溶预报中的应用[J].现代隧道技术,2011,48(5):76-81. [4] 李建朋,聂庆科,刘泉声,等.唐山市岩溶地面塌陷稳定性评价的Logistic回归模型[J].岩土力学,2017,38(S2):250-256. [5] 王瑞杰.三维地震波形差异属性分析在预测岩溶裂隙发育带中应用[J].中国煤炭地质,2018,30(1):72-75,80. [6] 袁永才,李术才,李利平,等.岩溶隧道施工过程中大型溶洞的综合预报及治理方案研究[J].现代隧道技术,2015,52(2):192-197. [7] 刘振华,范宏运,朱宇泽,等.基于BP神经网络的溶洞规模预测及应用[J].中国岩溶,2018,37(1):139-145. [8] Matthias S. Gaussian processes for machine learning [J]. International Journal of Neural System, 2004, 14(2): 69-106. [9] Mark G, Rogers S. Variational bayesian multinomial probit regression with Gaussian process priors [J]. Neural Computation, 2006, 18(8): 1790-1817. [10] Sofiane B, Bermak A. Gaussian process for nonstationary times Series Prediction[J]. Computational Statistics & Data Analysis, 2004, 47(4): 705-712. [11] 张研, 苏国韶, 燕柳斌.基于高斯过程机器学习方法的隧道围岩分类模型[J].现代隧道技术,2011, 48(6): 32-37. [12] 燕柳斌,张研,苏国韶.岩溶塌陷预测的高斯过程机器学习模型[J].广西大学学报(自然科学版),2011, 36(1): 172-176. [13] 贺建军,张俊星,贾思齐,等. 一种新高斯过程分类算法[J].控制与决策,2014,29(9):1587-1592 [14] Perrin G, Soize C, Marque-pucheu S, et al. Nested polynomial trends for the improvement of Gaussian process-based predictors[J]. Journal of Computational Physics, 2017, 346(1): 389-402. [15] Richardson R R, Osborne M A, Howey D A. Gaussian process regression for forecasting battery state of health[J]. Journal of Power Sources, 2017, 357(31):209-219. -
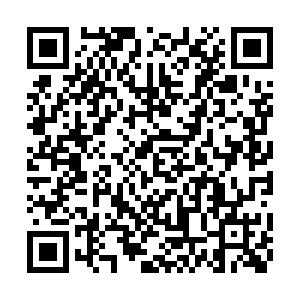
计量
- 文章访问数: 1560
- HTML浏览量: 640
- PDF下载量: 179
- 被引次数: 0