Evaluation of soil environmental quality in karst mountain area based on support vector machine: A case study of a tea plantation in northern Guizhou
-
摘要: 以贵州北部一茶叶园区80个表层土壤样品为研究对象,对其Hg、As、Cd、Pb、Cr和Cu含量进行测定,在MATLAB中应用支持向量机构建土壤环境质量评价模型,并与模糊综合评价法和内梅罗综合污染指数法的评价结果对比分析,探究支持向量机模型在喀斯特山区土壤环境质量评价中的适用性,其结果表明:研究区土壤质量Ⅰ类与Ⅱ类样品比例为33∶7,土壤环境质量大多数为I类;支持向量机方法的评价结果与模糊综合评价法和内梅罗综合污染指数法结果的相同率分别达到82.5%和80.0%,并分析结果有差异的样品,发现支持向量机评价结果更符合实际情况,这说明该模型适用于土壤环境质量的评价。Abstract: The content of heavy metals in soil directly affects the quality and safety of tea, and even has a potential threat to human health. It is hence important to monitor, evaluate and control the content of heavy metals in the tea plantation soil. In this paper, we select a tea plantation in karst mountain area of northern Guizhou as a study area. The area is located in the transitional zone from Yun-Gui Plateau to Hunan hilly area, which belongs to the humid monsoon region of tropical plateau, with the annual precipitation of 1,000 -1,300 mm and the annual average temperature of 12.6-13.1 ℃. In this study area, because the Cambrian and Ordovician carbonate strata are widely exposed, karst landform is well developed and is characterised by interlacing occurrence of peak clusters and karst valleys. According to present situation and the characteristics of land use, in the area, 80 surface soil samples were collected for the analyses of heavy metal (such as mercury (Hg), arsenic (As), cadmium (Cd), lead (Pb), chromium (Cr) and copper (Cu)) contents and the environmental quality of the tea plantation soil. To classify and evaluate the sample analytical results, the Support Vector Machine (SVM) model coded in MATLAB was employed. Meanwhile, by comparing the result from Nemerow comprehensive pollution index method with that of fuzzy comprehensive evaluation method, the applicability of SVM in soil heavy metal pollution evaluation was discussed. These results show that,(1) There are significant spatial differences in soil heavy metal contents, with the variation coefficients in the order from high to low of Cr>Hg>Cu>As>Pb>Cd. By comparing the average value of evaluation factors with the soil background value of Guizhou Province, it is found that the values of Cd and Cu are lower than the soil background values, and the others fall in between the background values and the secondary standard values of the national soil quality standard. In fact, the chemical concentrations of 91.25% of the soil samples are below the standard limits for tea producing areas, which represents a soil environment of non-pollution and high-quality for tea plantation.(2) The quality of soil in the study area is good, with its soil environmental quality ranging between category I and II.The evaluation results of SVM method are quite similar to those of fuzzy comprehensive evaluation and Nemerow comprehensive pollution index methods, with a similarity of 82.5% and 80.0%, respectively. During the application of these methods it was found that the results of SVM were more accurate, which showed that the model is suitable for the evaluation of soil environmental quality in karst mountain area. (3)In addition, the SVM can solve complex nonlinear problems, with much easier manual operation and less artificial intervention, comparing with the application of traditional assessment models. It provides a new idea and method for the evaluation of soil environmental quality in karst mountain area.
-
Key words:
- karst mountain area /
- Support Vector Machine /
- soil environmental quality
-
[1] 汪庆华,刘新.浅谈我国茶叶质量安全现状及应对措施[J].茶叶,2006,32(2):66-69. [2] 何璐君.开阳县茶园土壤重金属元素含量及污染评价[J].贵州农业科学, 2009,37(4): 184-186. [3] 李云,张进忠,童华荣.茶园土壤和茶叶中重金属的监测与污染评价[J].环境科学与技术,2008,31(5):77-81. [4] 李名升,张建辉,梁念,等.常用水环境质量评价方法分析与比较[J].地理科学进展,2012,31(5):617-624. [5] 邓琴,吴迪,秦樊鑫,等.岩溶铅锌矿区土壤重金属污染特征[J].中国岩溶,2017,36(2):248-254. [6] 柴世伟,温琰茂,张亚雷,等.广州市郊区农业土壤重金属污染评价分析[J].环境科学研究,2006,19(4):138-142. [7] 丁立,田林亚,范雷刚,等.基于灰色聚类法和灰色关联分析的大坝安全评价[J].测绘工程,2015,24(4):66-69,73. [8] 孙小涛,周忠发,黄智灵,等.改进模糊综合评价模型对岩溶山区茶叶产地土壤重金属污染评判[J].中国岩溶,2016,35(3):282-290. [9] 孟宪林,郭威.改进层次分析法在土壤重金属污染评价中的应用[J].环境保护科学,2001,27(1):34-36. [10] 任坤, 陈志兵, 潘晓东,等. 重庆南山老龙洞地下河表层沉积物重金属环境地球化学特征及生态风险评价[J]. 中国岩溶, 2016, 35(2):144-152. [11] 杨忠平,卢文喜,龙玉桥.改进BP算法在城市土壤环境质量评价模型的应用[J].重庆大学学报(自然科学版),2010,32(2):99-103. [12] 李晓婷,刘勇,王平.基于支持向量机的城市土壤重金属污染评价[J].生态环境学报,2014,23(8): 1359-1365. [13] 刘德地,陈晓宏.基于支持向量机的洪水灾情综合评价模型[J].长江流域资源与环境,2008,17(3):490-494. [14] 张成成,沈爱春,张晓晴,等.应用支持向量机评价太湖富营养化状态[J].生态学报,2013,33(23):7563-7569. [15] 张锦水,何春阳,潘耀忠,等.基于SVM的多源信息复合的高空间分辨率遥感数据分类研究[J].遥感学报,2006,10(1):49-57. [16] 周建国,张希刚.基于粗糙集与灰色SVM的中国CO2排放量预测[J].中国环境科学,2013,33(12):2157-2163. [17] Yoon H, Jun S C, Hyun Y, et al. A comparative study of artificial neural networks and support vector machines for predicting groundwater levels in a coastal aquifer[J]. Journal of Hydrology, 2011, 396(1):128-138. [18] 梁雪春,龚艳冰,肖迪.一种多核加权支持向量机的水质预测方法[J].东南大学学报:自然科学版,2011,41(S1):14-17. [19] 姜雪,卢文喜,杨青春,等.应用支持向量机评价土壤环境质量[J].中国环境科学,2014,34(5):1229-1235. [20] 陈其松.智能优化支持向量机预测算法及应用研究[D].贵阳:贵州大学,2009. [21] Vapnik V N. The nature of statistical learning theory[M].Berlin: Springer-Verlag,1995. [22] 张强,黄生志,陈晓宏.基于支持向量机的土壤湿度模拟及预测研究[J].土壤学报,2013, 50(1):59-67. [23] LI Wei-Xin,ZHANG Xu-Xiang,WU Bing,et al.A Comparative Analysis of Environmental Quality Assessment Methods for Heavy Metal-Contaminated Soils[J]. PEDOSPHERE, 2008, 18(3):344-352. [24] 梁伟,张慧颖,朱孔来.基于模糊数学和灰色理论的城市生态环境竞争力评价[J].中国环境科学,2013,33(5):945-951. [25] Swaine D J. Why trace elements are important[J]. Fuel Processing Technology, 2000, 65:21-33. [26] 国家环境保护总局.土壤环境监测技术规范:HJ/T166-2004[S].北京:中国环境科学出版社,2004. [27] 刘红艳,周燕,王铁夫,等.ICP-MS法测土壤样品中铜铅的不确定度评定[J].光谱学与光谱分析,2011,31(4):1115-1118. [28] Wilding L P. Spatial variability: Its documentation, accommodation, and implication to soil surveys[M]//NIELSEN D R, BOUMA J. Soil Spatial Variability. Wageningen: Pudoc, 1985: 166-194. -
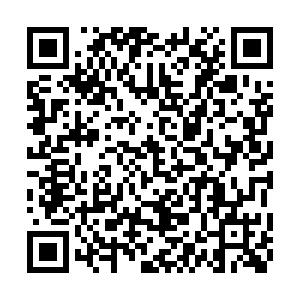
计量
- 文章访问数: 2042
- HTML浏览量: 561
- PDF下载量: 663
- 被引次数: 0